ATTENTION NEWS JUNKIES: We are the new Drudge, be sure to check our news feed by CLICKING HERE
FOLLOW REVOLVER CONTRIBUTOR DARREN BEATTIE ON PARLER
This guest post is the third installment of Revolver News’s ongoing series on election fraud. Revolver News is applying a rigorous, statistically informed approach to investigating fraud in the 2020 United States presidential election. We encourage the reader to consult Part 1 and Part 2 of the series, as well as our summary of another major statistical analysis.
Guest Post by Carl Bell
Short Summary:
We construct a new metric of potential voter fraud using suspicious distributions of birthdays in Pennsylvania voter registration data. The basic idea is that people picking fake birthdays will make predictable non-random choices, like picking round numbers for days of the month, and not knowing what true birth month distributions look like.
Under this metric, a number of counties in Pennsylvania have extremely unlikely distributions of voter birthdays. Seven counties representing almost 1.4 million votes total (Northumberland, Delaware, Montgomery, Lawrence, Dauphin, LeHigh, and Luzerne) have suspicious birthdays above the 99.5th percentile of plausible distributions, even when using conservative assumptions about what these distributions should look like.
These suspicious birthdays also matter significantly for election outcomes. While there are suspicious counties that vote Republican overall, in general more suspicious birthdays in a county are strongly associated with a larger Biden vote share, and a higher Biden vote share relative to all Democrat presidential candidates since 2000. More suspicious birthdays are also associated with a higher vote share for Jorgensen relative to Trump (consistent with a fraud scheme aiming to get Biden high but not “too high”, while simultaneously giving as few votes to Trump as possible).
Finally, we quantify the magnitude of how this potential fraud may have impacted the election. Even a small reduction in the amount of suspicious birthdays (to the 98th percentile of the conservative distribution) would be predicted to have resulted in Trump winning the state by 71,500 votes. This suggests that whatever is driving the anomalous patterns in birthdays is sufficiently important to affect the statewide election result.
Executive Summary:
We use a largely ignored data source to identify suspicious voter registrations by county, a data source that is independent of the actual vote outcomes. In other words, we will construct metrics that identify counties that show indications of potential voter fraud regardless of who a county is voting for. Then, once this is done, we will show how these measures correlate with vote outcomes.
Our key insight is that someone making up fake birthdays for voter registrations is unlikely to be able to do so in a truly random manner. Instead, we identify several likely hallmarks of fake birthdays:
-They are likely to excessively cluster on round number days of the month (1, 10, 15, 20, 30, 31), since people generally overweight round numbers.
-They are likely to excessively cluster on January and December for the same reason.
-They are likely to excessively cluster on months of the year which in general have few birthdays in overall demographic data (i.e. fake birthdays will be drawn roughly evenly across months, subject to the round number effect above, while true birthdays tend to cluster more in certain months like July and August, and less in months like February and November).
We call these “suspicious birthdays” — individually any one person can easily have any of the traits above, but having too many overall in a county suggests that fake birthdays have been added to the pool. We take these three measures of suspicious birthdays, and evaluate them against a combination of two types of benchmarks of what might be expected in the absence of fraud. These are designed to ensure that any unusual patterns are not coming from other reasons (e.g. births generally avoiding holidays, or people generally having sex more at certain times of the year):
-A “best guess” benchmark, where we compare each county to overall demographic data:
Historical Day-of-the-month in birthdays from the Social Security Master Death File, and
Historical Month-of-the-year in birthdays for that county from the National Center for Health Statistics
-A “conservative” benchmark, where we also add in measures of each county relative to the distribution of all voter birthdays in the state of Pennsylvania. This has the effect of measuring how unusual each county looks just compared to other counties, and so effectively strips out the average level of fraud across all counties.
We find that even under the conservative distribution, seven counties representing almost 1.4 million votes total (Northumberland, Delaware, Montgomery, Lawrence, Dauphin, LeHigh, and Luzerne) have numbers of suspicious birthdays above the 99.5th percentile of plausible distributions. This represents the average abnormal metric across six different ways of measuring suspicious birthdays. In other words, these counties are not abnormal just along one or two measures, but across the whole range of them. The three worst offenders, Northumberland, Delaware and Montgomery, are above the 99.97th percentile, the 99.91th percentile, and 99.74th percentile respectively, a result extremely unlikely to occur by chance. Montgomery also has significant evidence of voter fraud across entirely separate measures. Meanwhile, 15 counties score above the 95th percentile of abnormal birthdays on average, and these represent almost 3.5 million votes (the additional eight are Berks, Northampton, Cumberland, Bucks, Philadelphia, Monroe, Lancaster and Erie). Recall, these measures are under the conservative benchmark – under the best guess benchmark, the deviations look even more extreme.
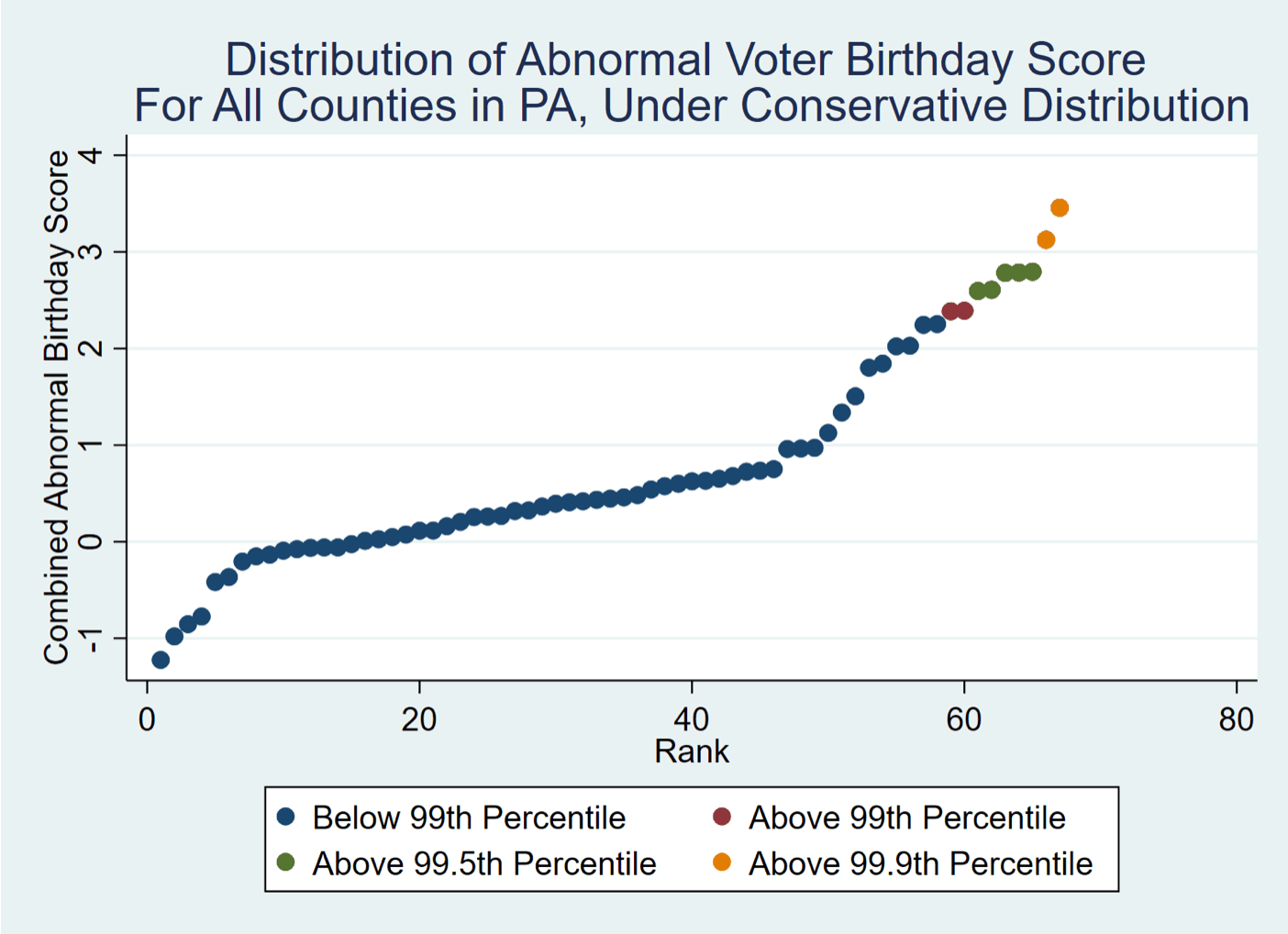
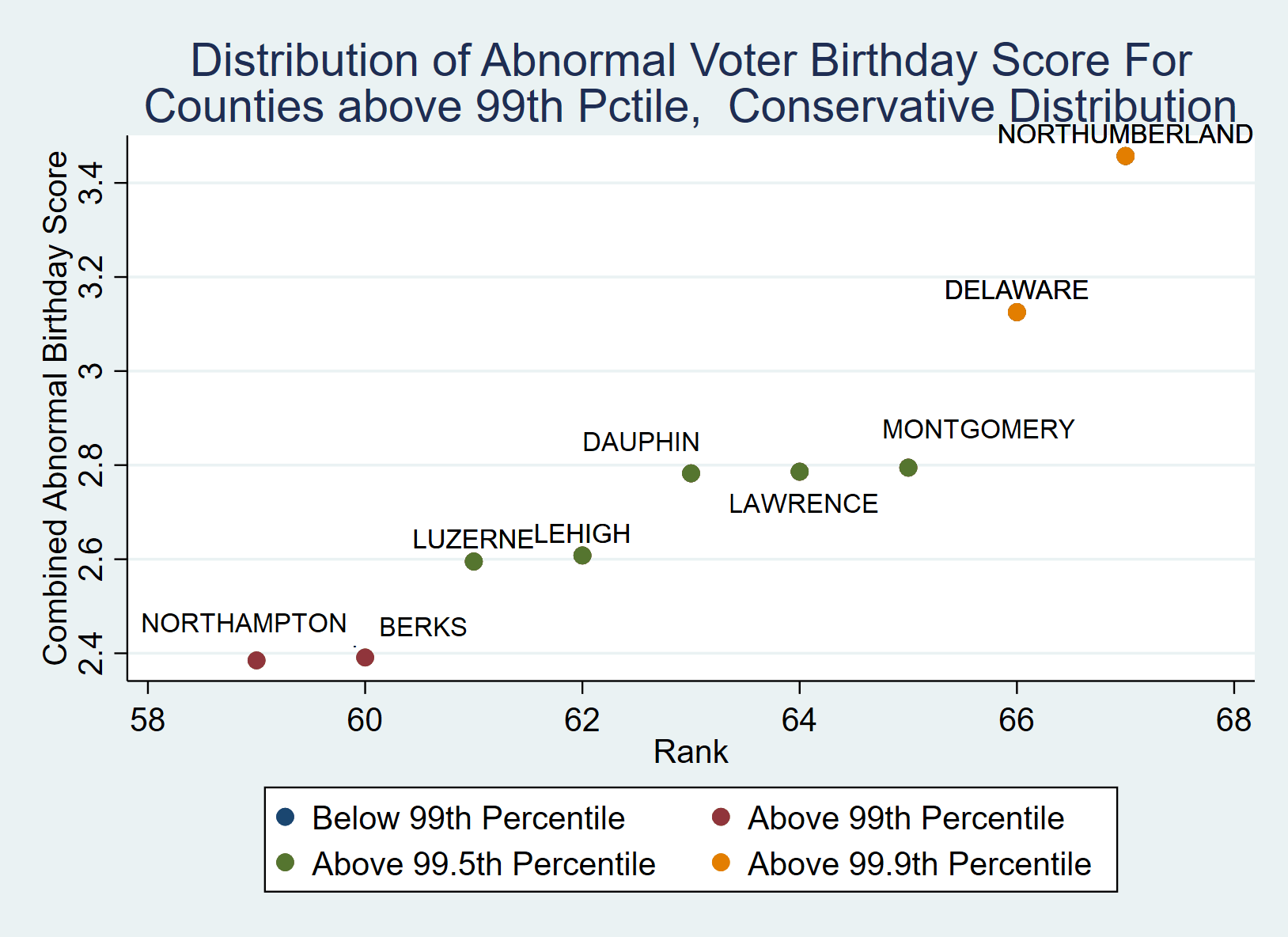
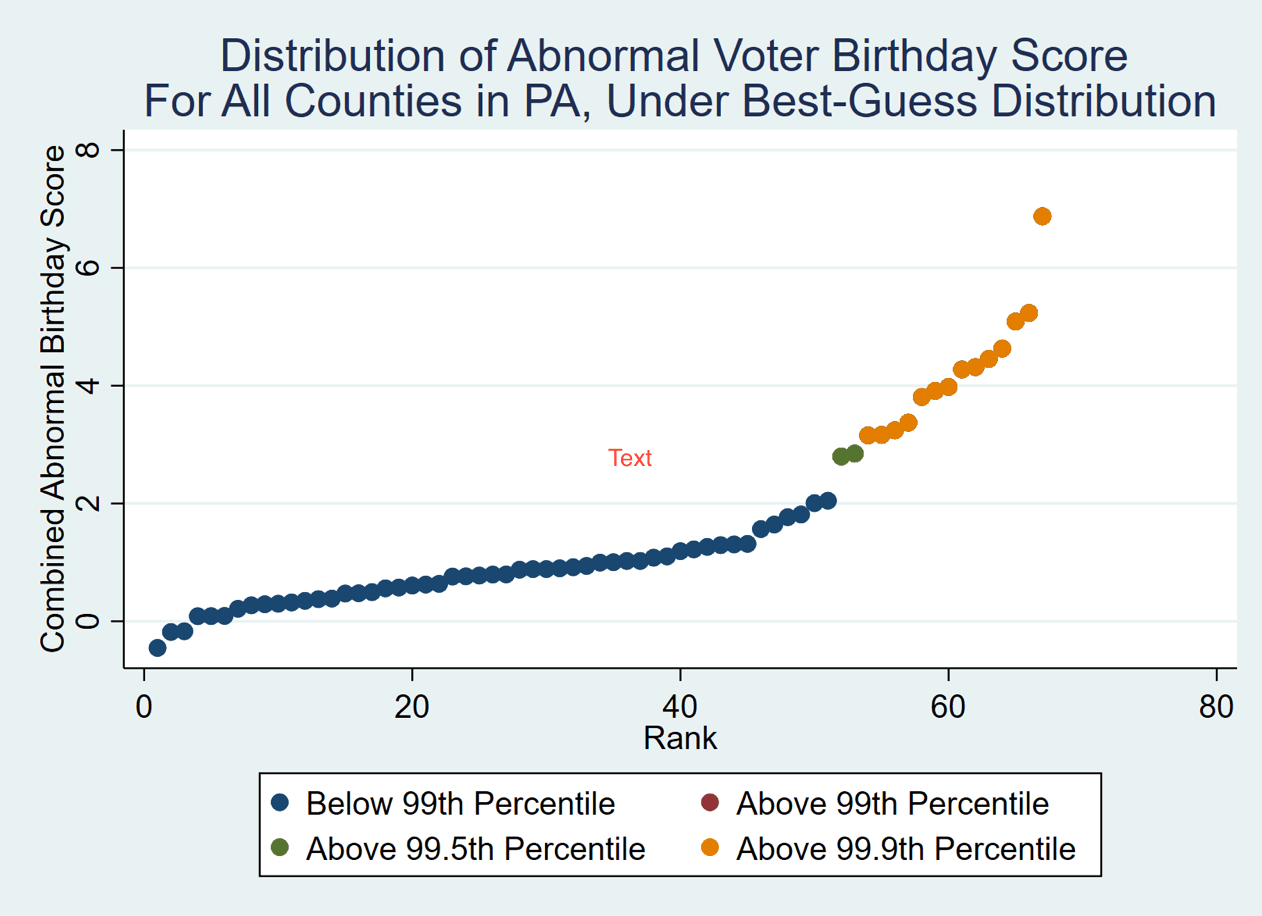
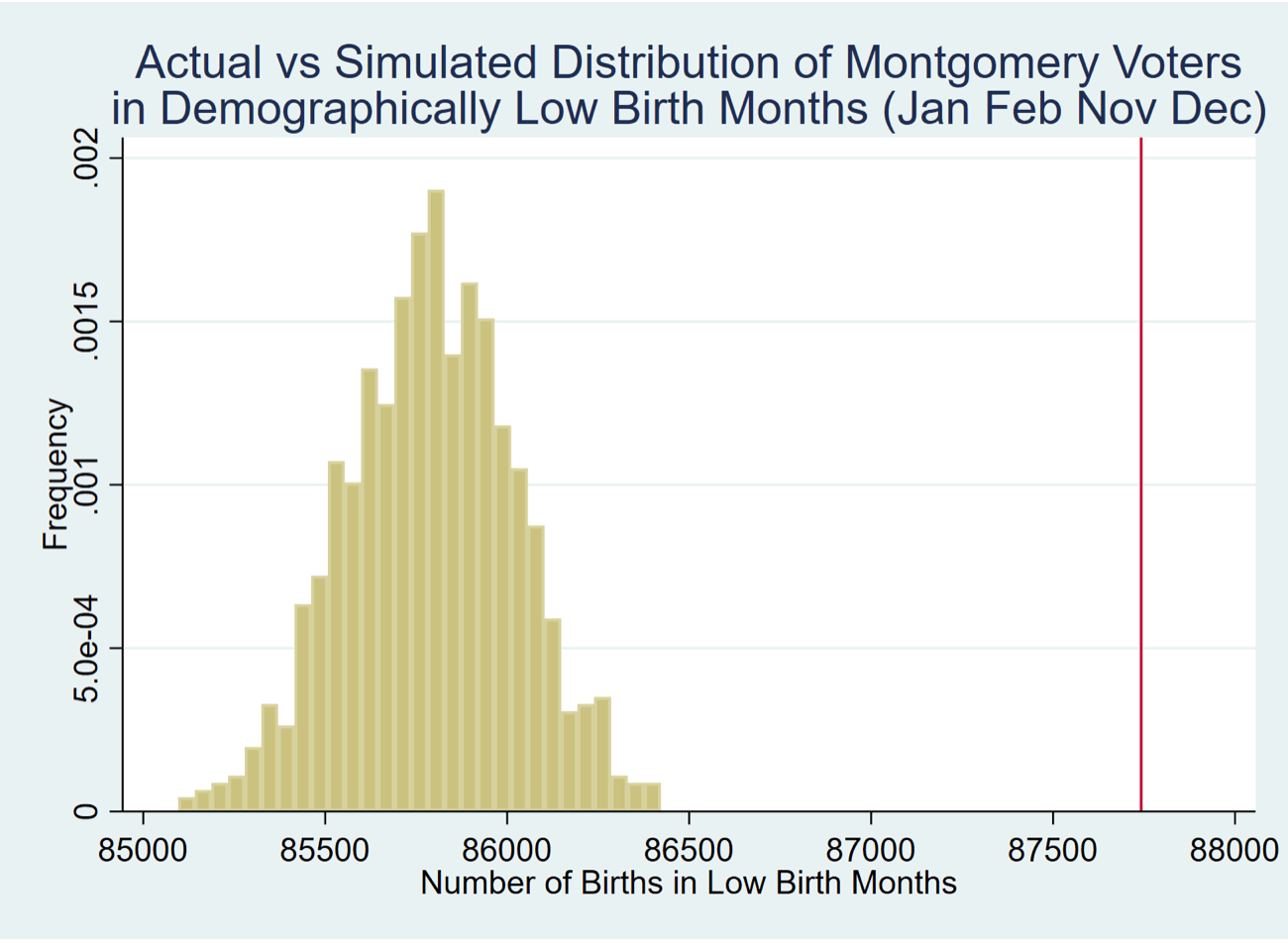
Next, having identified measures that indicate potential voter fraud, we show that they also make a large difference to county election outcomes. A greater level of suspicious birthdays in a county is significantly related to higher vote share for Biden. A one standard deviation increase in suspicious birthdays is associated with a 6.8 percentage point increase in the two-party vote share for Biden. The probability of observing such a relationship by chance (i.e. the p-value) is less than 0.000008.
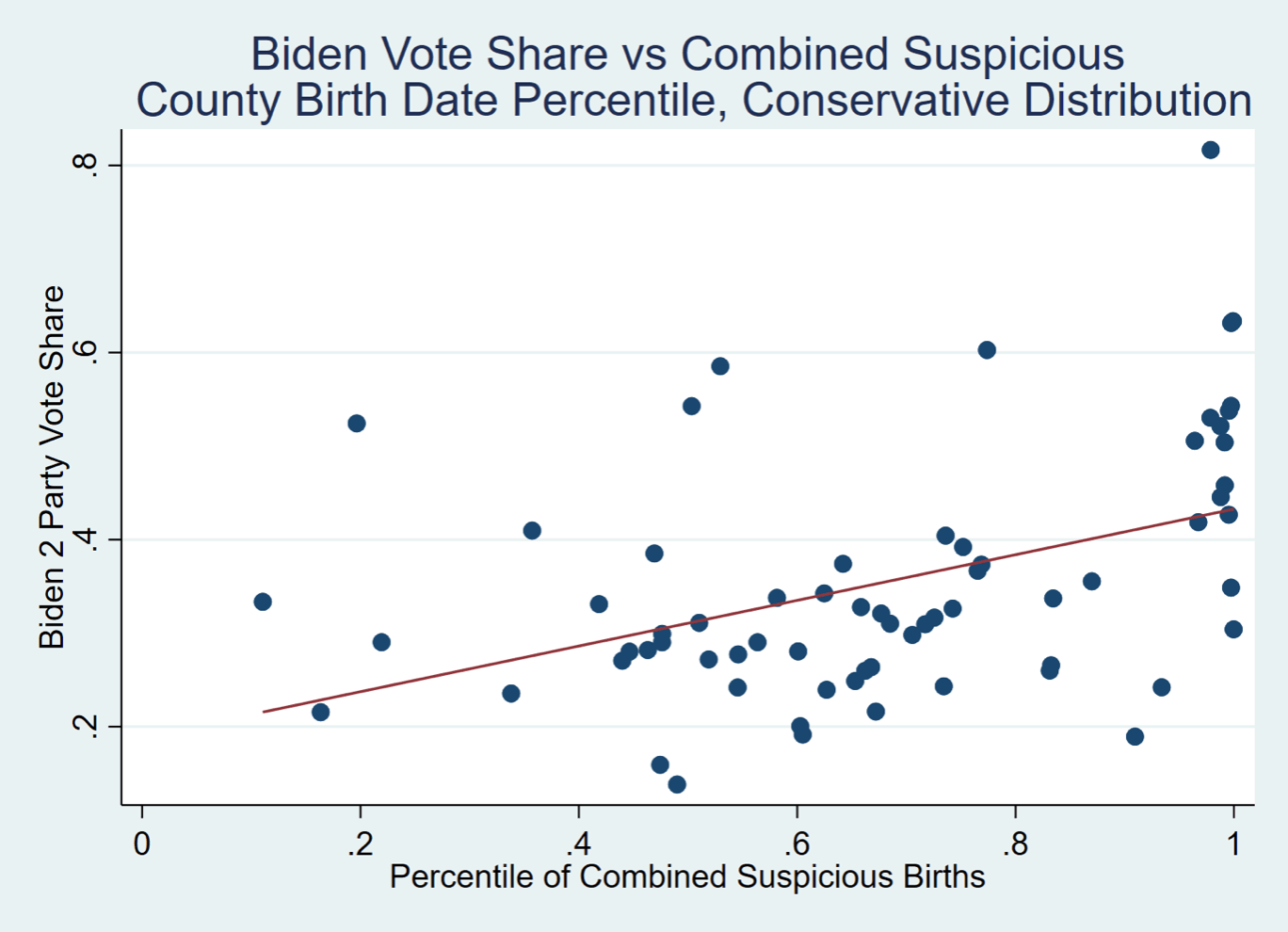
It is worth noting that abnormal counties are not exclusively majority Democrat – indeed, the most extreme county in suspicious birthdays, Northumberland, voted almost 70% for Trump. Lawrence, Luzerne and Berks, also majority Republican, look suspicious as well. This is consistent with a genuine measure of fraud – it would be highly surprising if fraud were a uniquely Democrat phenomenon.
Nonetheless, higher likelihood of fraud is strongly associated with more votes for Biden. Out of the 13 counties in Pennsylvania who voted majority Biden, 9 are above the 95th percentile of suspicious birthdays.
Second, we show that more suspicious birthdays are also associated with a county having a higher presidential Democrat vote share relative to all previous elections in recent history. The p-value for this relation is less than 0.003, and a one standard deviation in abnormal birthdays increases Biden’s vote share relative to all recent past elections by 2.4 percentage points. There are 5 counties out of 67 where Biden’s two-party vote share exceeded the performance of the Democratic candidate in all presidential elections since 2000 – Montgomery, Delaware, Cumberland, Allegheny, and Chester. Of these, three are above the 98th percentile of the suspicious birthday distribution.
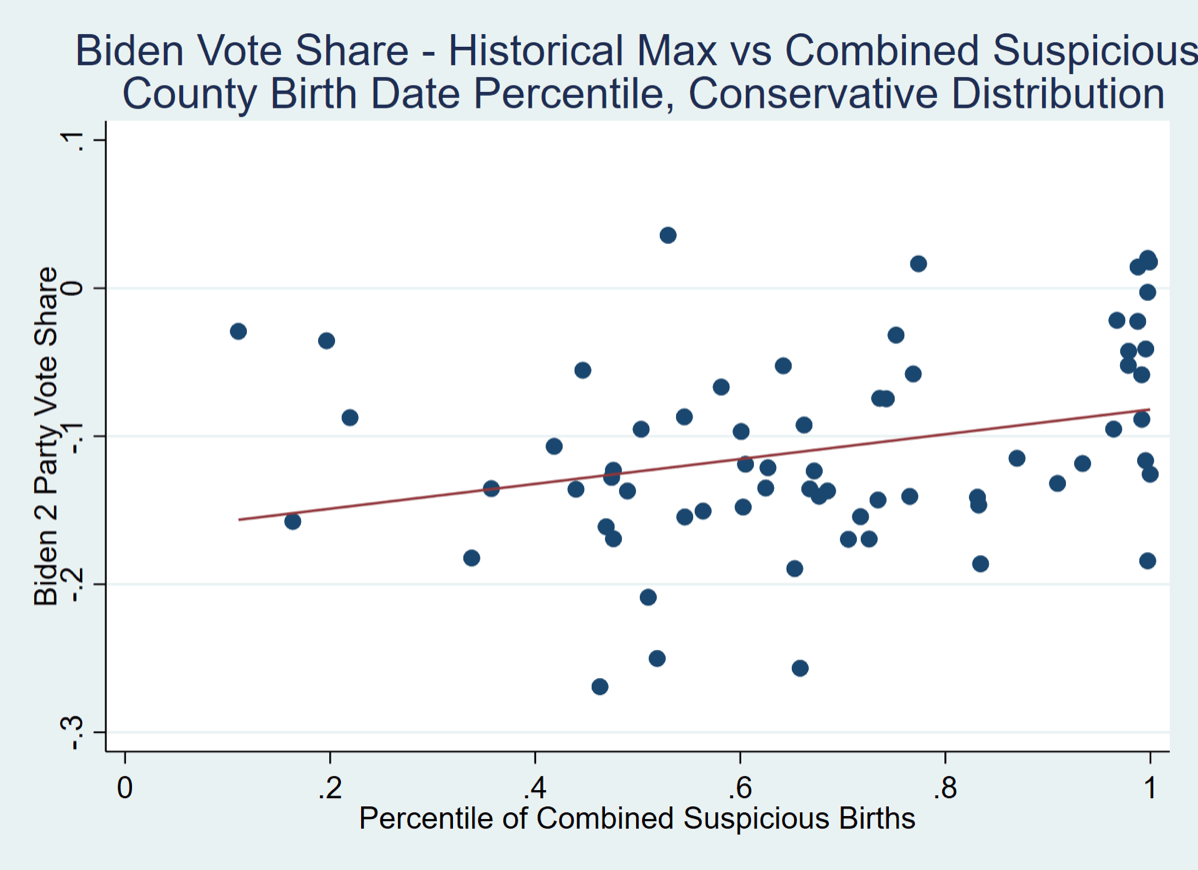
Third, more suspicious birthdays are also associated with higher vote shares for Jorgensen relative to Trump. This is an additional likely consequence of fraud – if a perpetrator wants to maximize the overall contribution to Biden over Trump in the statewide race, and doesn’t want to report an implausibly high overall vote for Biden, the only alternative is to add votes to Jorgensen.
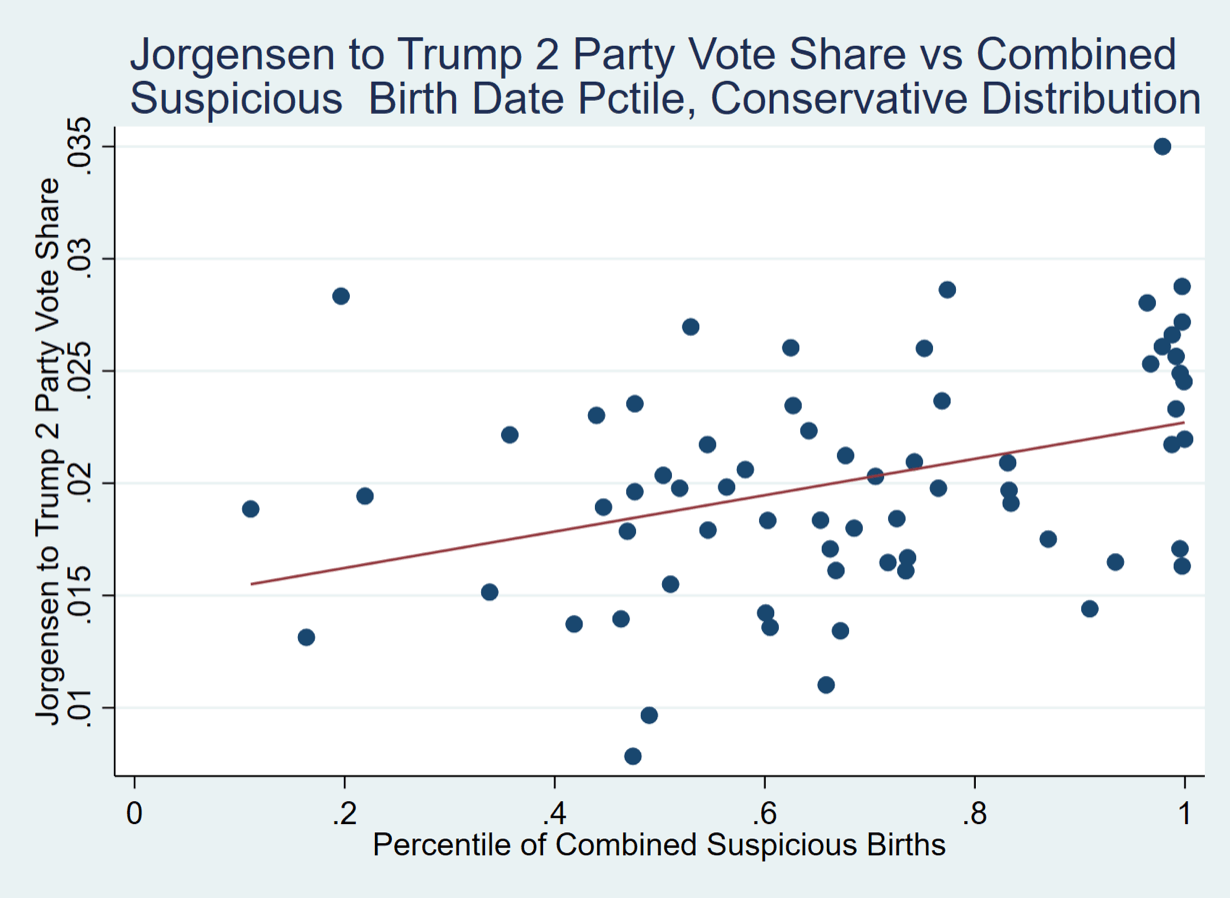
Finally, we can use these results to estimate the likely effect of suspicious birthdays on the overall Pennsylvania election outcome. Because someone making up birthdays will not always select, for example, round days in January or December, the actual numbers of excess suspicious birthdays are likely to considerably understate the magnitude of possible fraud. As a result, we use the relationship between excess birthdays and Biden vote share to estimate the effect of a change in the magnitude of suspicious births on county vote outcomes.
In particular, we consider what would happen if the ten counties who scored above the 98th percentile of suspicious birthdays under the conservative distribution were instead to merely be exactly at the 98th percentile. This would still leave these counties looking very suspicious, but merely less so. Even this minor change would result in an additional predicted 76,600 votes for Trump and the same number fewer for Biden, which would be enough to swing the state to a Trump win overall by 71,500 (as Biden has a vote lead of 81,660 votes).
These results suggest strongly the presence of abnormal birthday distributions consistent with a large number of fraudulent voter registrations. They also provide strong evidence that the presence of such abnormal birthdays is positively associated with more votes for Biden, including at historically anomalous levels. Finally, the magnitude of these suspicious birthdays is plausibly large enough to affect the entire statewide outcome of the Pennsylvania presidential election vote.
Detailed Description of Analysis
Data Sources
Pennsylvania Voter Data, the Social Security Master Death File, and the NYT county election results are all available at the “Audit the Election” wiki:
https://wiki.audittheelection.com/index.php/Datasets
County birth data is available from the National Center for Health Statistics
https://www.cdc.gov/nchs/data_access/vitalstatsonline.htm
Definition of Suspicious Birthday Scores
We will start with a definition of what we mean by suspicious birthdays, and how we measure them. To construct each score of suspicious birthdays, we need two aspects:
- A definition of the measure we expect to be higher in a list of fictitious/fraudulent birthdays
- A benchmark for what we expect this number to be absent fraud.
Once we have each combination of measure and benchmark, our strategy is to take each county and simulate 1000 random draws of all the voters in that county from the benchmark distribution. This tells us how wide the range of variation is that we might expect to find just by chance. Then, we take the actual value in the true data, and subtract off the mean of the benchmark distribution, and divide by the standard deviation of the benchmark distribution. This produces what is known in statistics as a z-score for that county and metric – in other words, how many standard deviations away from the simulated mean the true value is.
The z-score has several desirable properties. First, it gives a measure of the magnitude of the anomaly that is comparable across counties of different sizes, as each one is compared to the range of expected variation for that county in the simulated distribution. Second, it can be averaged across multiple different anomalies for a given county, and retains the intuition of being an overall measure of how unusual the county looks across multiple dimensions. Third, the z-score (either for the individual anomaly, or the aggregated measure) can be turned into an associated probability under the normal distribution, which measures how unlikely the particular outcome is. This is particularly valuable for the aggregated anomaly measure, where it is not straightforward otherwise to simulate the joint outcome of all the anomalies together.
Below, we describe each of the six scores we generate per county, which are then averaged together to form the aggregate suspicious birthday score. We explain how each score arises from the combination of measures and benchmarks. The first three scores form the best-guess distribution, while the conservative distribution (which we use through most of the analysis) adds the final three scores.
Three Scores Under Best Guess Distribution
Score 1: Round Day-of-Month, Measured Relative to Social Security Birthdates
Measure: Excess birthdays on round days of the month (1, 10, 15, 20, 30, 31)
Benchmark: Birthday distribution in Social Security master death file
In this measure, we hypothesize that someone making up birthdays will be likely to oversample suspiciously round numbers. We test this in the aspect that has the strongest intuitive reasons to be random and uniform across counties – the day of the month on which the person was born. While there are strong reasons why births may cluster on particular days of the week (such as scheduled C-sections avoiding weekends), it is not clear why the distribution of days within a month should vary across counties.
Rather than simply assume uniformity in day-of-birth within a given month (which may be considered an intuitive benchmark), we instead benchmark each county to the overall distribution of day-of-the-month for birthdays in the Social Security Master Death File, for births after 1940. Getting administrative data on the distribution of day-of-the-month for birthdates is surprisingly hard – it is not contained in the American Community Survey (which only gives birth quarter), nor the National Center for Health Statistics births file (which only gives birth month, starting in 1989). The reason is that that exact birthdays often are important personal identifying information, and so are not included in many administrative datasets (except for dead people, where the issue is less important). This distribution is only for those already dead and so is thus somewhat selective, and not available at local levels. Nonetheless, it is the best publicly available metric for actual days of the month in a real, national sample of births.
Score 2: January and December Births, Relative to County Historical Data
The second main measure we use is an overweighting of January and December birthdays. For someone picking round numbers, the first and last month are likely clusters. This is the equivalent of the 1st/31st days above. As it turns out, January and December are also demographically low birth months (the measure used for score 3), so these are actually doubly likely to be overweighted. Recall that we are not predicting that January and December should have more birthdays overall than other months, because the overall voter distribution will be made up of a large mass of genuine voters (who will underweight January and December for demographic reasons) plus a likely smaller mass of fraudulent voters who will overweight these months. As a result, the estimate is that there will be too many relative to the base guess of the low numbers in the benchmark distributions.
As a first benchmark, we take the distribution of months for all births within that county between 1989 and 2002, from the National Center for Health Statistics. For 31 larger counties in Pennsylvania, county level breakdowns are given, and for these counties we use the county-specific distribution. For the remainder, we use the average distribution across all small counties (which are not separated in the NCHS data). The series we use starts only in 1989, as before this time, only the birth year was reported. Nonetheless, this gives a measure for the range of birth months for everyone born in that particular county for 14 years of eligible voter birthdays.
Score 3: Demographically Low Birth Months, Relative to County Historical Data
The third main measure we use is the presence of too many birthdays in months that demographically have fewer births. The base idea here is that someone making up birthdays will be roughly randomizing across months (subject to the tendency to overweight January and December as round numbers). By contrast, real birthdays tend to cluster in certain months of the year. In Pennsylvania, the months with historically low birthdates are January, February, November and December (all these months are below the 8.33% rate under a uniform distribution, for all 31 counties and for all 14 years of data). If one picks a month at random, one will end up with too many birthdays from these demographically “low birth” months, and not enough birthdays from the demographically high birth months. The two are mirror images, but to keep the prediction in the same direction (i.e. too many birthdays of type X) we test the number of birthdays in demographically low birth months.
In the first benchmark, we compare this again with the same-county historical births data for the 31 counties with detailed data, and with the remaining county average for all rest. For each county distribution, because we have the history of 14 years of birth dates, we take the measure of “low birth” months from the overall average of all births in the county over those 14 years. That is to say, while low birth months show a lot of overlap (e.g. always including Jan, Feb, Nov and Dec), we allow the distribution to vary by county, to allow maximum flexibility for what we should expect to see in that particular county.
Additional Metrics for Conservative Distribution
For scores 4, 5 and 6, we take the same three metrics above, and we also examine how each county scores relative to the benchmark of all county voter registration birthdays. In essence, it simply measures which counties look unusual relative to other counties, without taking any stand on what the underlying distribution should be. This has the advantage that it takes into account any possible odd selection effects that may be present around which types of birthdays register to vote. However, it is likely to understate the magnitude of possible fraud, by effectively stripping out the average level of fraud from the distribution. In other words, suppose hypothetically birthdays were made up of 75% honest counties (with almost uniform distributions of days) and 25% fraudulent counties (with excess round numbers). The average frequency of round numbers would be higher than uniform due to fraud, but this benchmark would strip out the average effect, and just identify counties that looked more fraudulent than the average.
By averaging these together (along with the other scores), we thus bias ourselves against finding overall measures of fraud, but also consider how unusual a county looks relative to other counties. We do this for all the three metrics above, using either the distribution of days of the month, or months of the year. For the low birth month measure, the only variation is that our definition of low birth months applies now uniformly across counties, so we take the four months that score low in every county and year observation – January, February, November and December. As before, these are benchmarked against all voter birthdays in Pennsylvania.
Effect of Aggregating Measures
This gives us either three or six z-score measures of abnormal birthdays for each county (depending on whether the conservative or best guess measure is used). Each z-scores measure effectively “how large are the deviations from what is expected”? Next, we can measure the probability of each outcome – effectively “how unlikely is the true data under the benchmark?”. For the statistically inclined – for individual scores, we can just use the percentile rank in the bootstrapped distribution, but for the aggregated measure, have to convert the average z-score to a probability using the normal distribution. In either case, we end up with an overall probability of observing an outcome as unusual as the one we actually see.
Having obtained each of the three or six measures, we average them for the county, and compute the associated probability of a number that extreme. This forms the basis for our subsequent tests. For simplicity, we will just refer to this as the “abnormal birthday score”, and the associated percentile in the distribution as the “abnormal birthday percentile”. Unless otherwise specified all results use the conservative distribution. The relations documented below generally get stronger if the best guess distribution is used instead.
It is worth noting what impact it has to aggregate all the measures. If there actually is fraud, then adding in more measures will improve our reliability – scoring high on lots of different metrics provides a stronger signal of fraud than just scoring high on one. By contrast, if any one of our measures is not actually a measure of fraud, then adding it will bias the average z-score towards zero, and will mean all counties will look less suspicious.
Second, our prediction actually has a specific direction. We are not measuring whether a county just looks unusual on a measure by being either too high or too low. Our predictions specifically require that a county scores highly, and when we average, we test if the county scores high on average across all the measures. If a county scores too low on one particular measure (e.g. it has too few birthdays in January and December, rather than too many), this might be considered suspicious in some ways, but in our measure, this will actually be considered anti-fraud – adding in a negative number to any existing positive numbers will bring down the average. In summary, adding in many metrics the way we do will actually bias us against finding results if some of our measures are incorrect.
Finally, it is worth noting that these distributions of unusual birthdays are inherently suspicious regardless of whether they predict any particular outcome. In other words, the basis for suspicion is not because these metrics predict outcomes. The basis for suspicion is that these metrics look highly implausible relative to what we would expect. The predictive power merely speaks to the question that these deviations are likely to be quantitatively important for affecting the election outcomes.
Possible Alternative Explanations
The metrics chosen are designed to represent patterns that are very plausible under fraud, but that do not have many obvious alternative explanations. That is to say, it seems plausible that these measures ought not vary much across counties, as a base assumption. Such an assumption would not be a good one for birth years, for instance, where there are strong reasons to think that some counties will have more young people than others. But it is not nearly as obvious why some counties should have more 10th of the month birthdays than other counties.
However, for each metric, alternatives are possible. Nonetheless, one of the other strengths of combining multiple metrics is that it also is much more difficult to explain the results merely with possible alternative explanations for one of the underlying components. Suppose one has a theory of why there are excessive births on round days of the month, for instance – maybe scheduled C-sections avoid certain holidays (though why there should be too many on New Years Eve, New Years Day, Martin Luther King Day etc. is another question). Such a theory would also have to explain not just the level of births being non-uniform (because, recall, this pattern in general ought to be in the Social Security birth data too). It would also need to explain why this pattern should vary across counties. Having done all that, it would further need to explain why such a pattern should predict so many various aspects of election results.
Now, suppose one has generated such an explanation. There is now the additional hurdle of explaining why there should also be too many birthdays in January. If one manages to do that, one further has to explain why there ought to also be too many birthdays in February and November. And one has to explain why both these facts vary across counties in this predictable manner. This becomes much harder.
Similar difficulties arise from the benchmark distributions. Suppose one doesn’t think the social security birthdays are the right benchmark, notwithstanding the difficulty of finding better ones. In that case, the metrics using voter birthdays as the benchmark do not rely on this data. Similarly, if one is worried about the historical birth data not being reflective of current populations, the voter data also does not predict the same problem should occur there. If one is worried that the voter birthdays may have innocent errors for reasons other than fraud, then using alternative benchmarks from administrative data sources circumvents this problem.
And recall, the hurdles of an alternative explanation are twofold. First, they must explain why the distribution looks so anomalous in the first place. That is to say, the level of the distribution looks wrong. And even if this is accomplished, they must also explain why these deviations should predict so many aspects of vote outcomes – not just Democrat performance, not just Democrat performance relative to all recent elections, but also the relative preference for Libertarians and Republicans.
We do not assert that such an alternative is impossible. But the nature of the combined metric makes it much more difficult to explain the overall pattern of results with any single criticism of one particular score.
Distribution of Abnormal Birthday Metrics
First, we compute the overall distribution of abnormal birthday scores under the conservative distribution of aggregating all six measures. We find that even under the conservative distribution, seven counties representing almost 1.4 million votes total (Northumberland, Delaware, Montgomery, Lawrence, Dauphin, LeHigh, and Luzerne) have numbers of suspicious birthdays above the 99.5th percentile of plausible distributions. This represents the average abnormal metric across six different ways of measuring suspicious birthdays. In other words, these counties are not abnormal just along one or two measures, but across the whole range of them. Meanwhile, 15 counties score above the 95th percentile of abnormal birthdays on average, and these represent almost 3.5 million votes. Recall, these measures are under the conservative benchmark – under the best guess benchmark, the deviations look even more extreme.
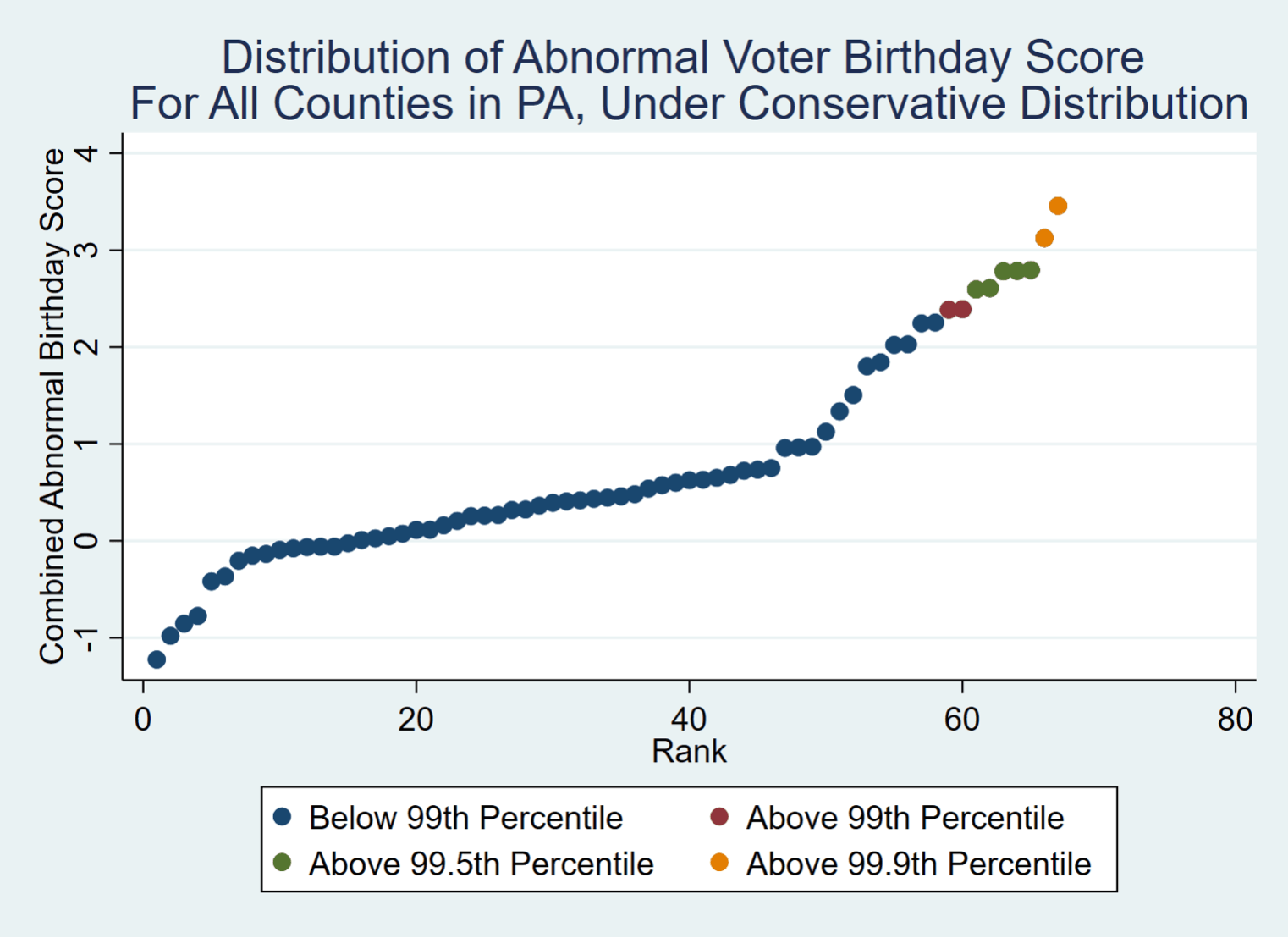
It is also worth observing the asymmetry in these z-scores. The lowest scores are negative, but not by very much. However, the highest scoring counties not only look much larger and positive, but do not fit the rest of the pattern of the curve.
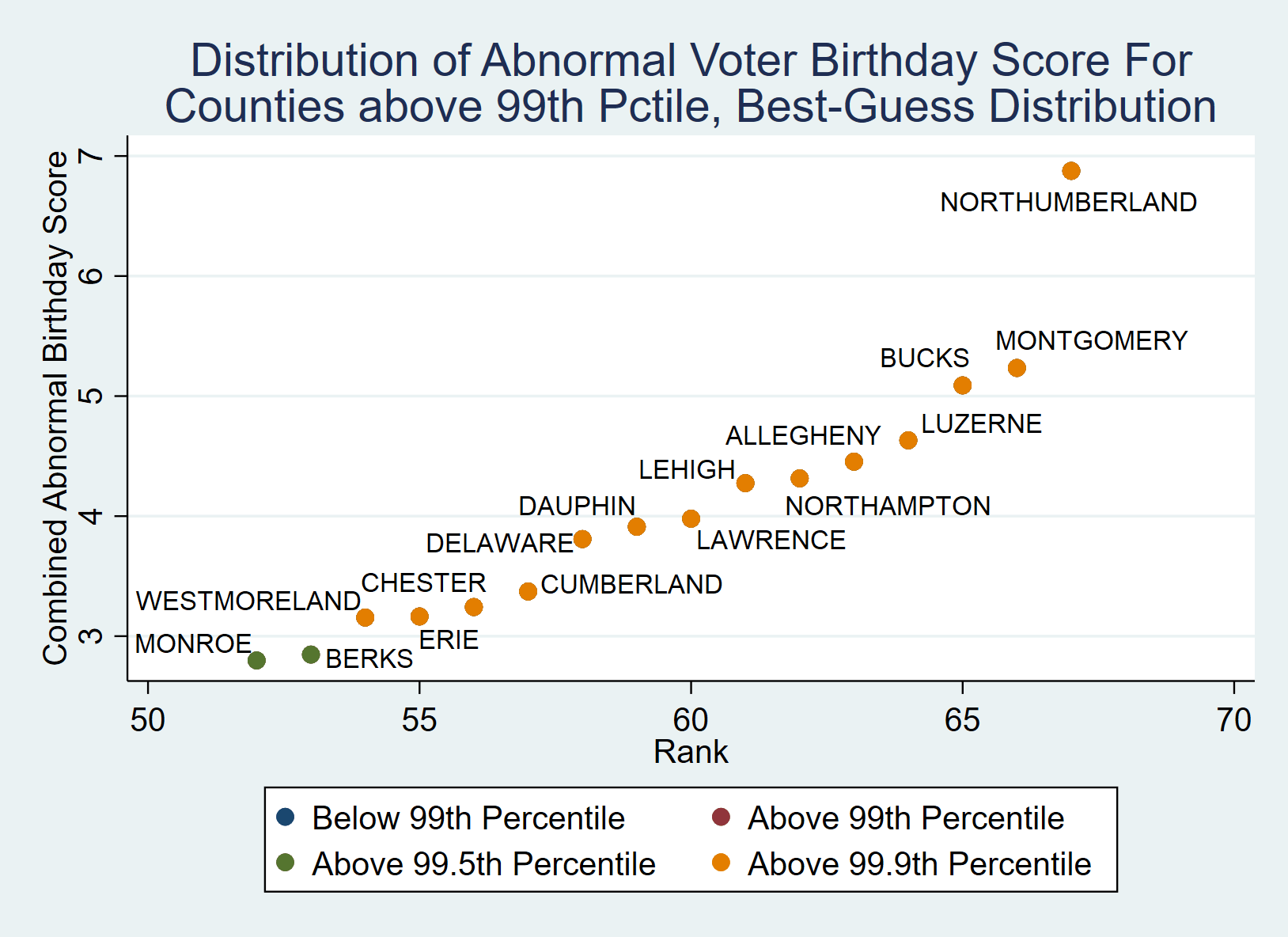
The three worst offenders, Northumberland, Delaware and Montgomery, are above the 99.97th percentile, the 99.91th percentile, and 99.74th percentile respectively, a result extremely unlikely to occur by chance. Montgomery also has significant evidence of voter fraud along entirely separate measures. To show just one example of the underlying simulations that go into the score, below is shown the simulated distribution for Montgomery of the likely number of births in low birth months (Jan, Feb, Nov, Dec) based on the historical birth data, and the actual value. As the graph shows, the county is a colossal outlier.
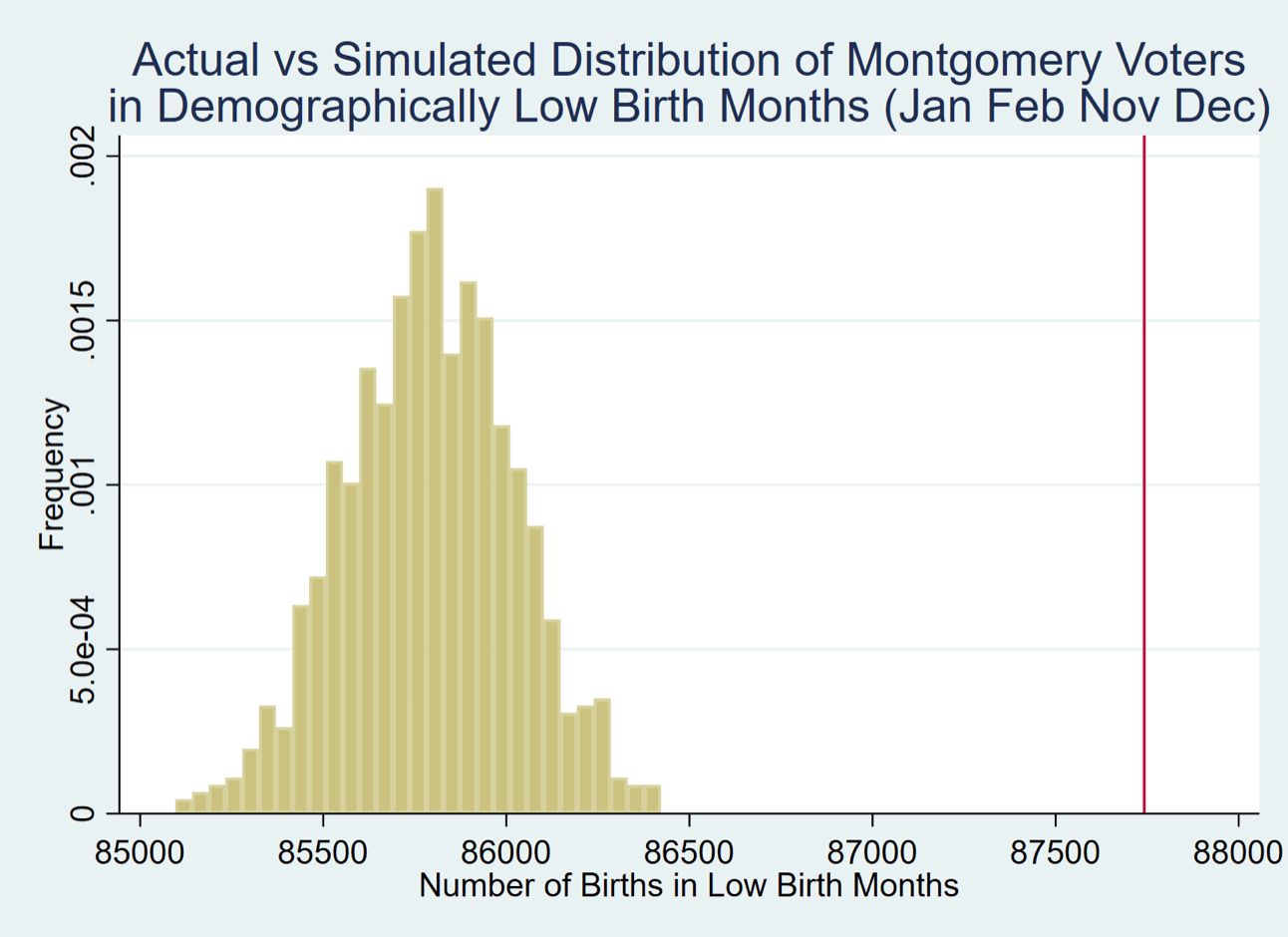
However, these graphs actually understate the likely extent of the problem, because three of the six scores (using the voter birthday distribution) effectively strip out the average level of fraud, by comparing only the distribution of voter birthdays, including any fraudulent ones. If we instead plot the best-guess distribution (which does not derive from the same voter birthdays being examined) the majority of the counties look fairly similar, while the outliers look even more extreme.
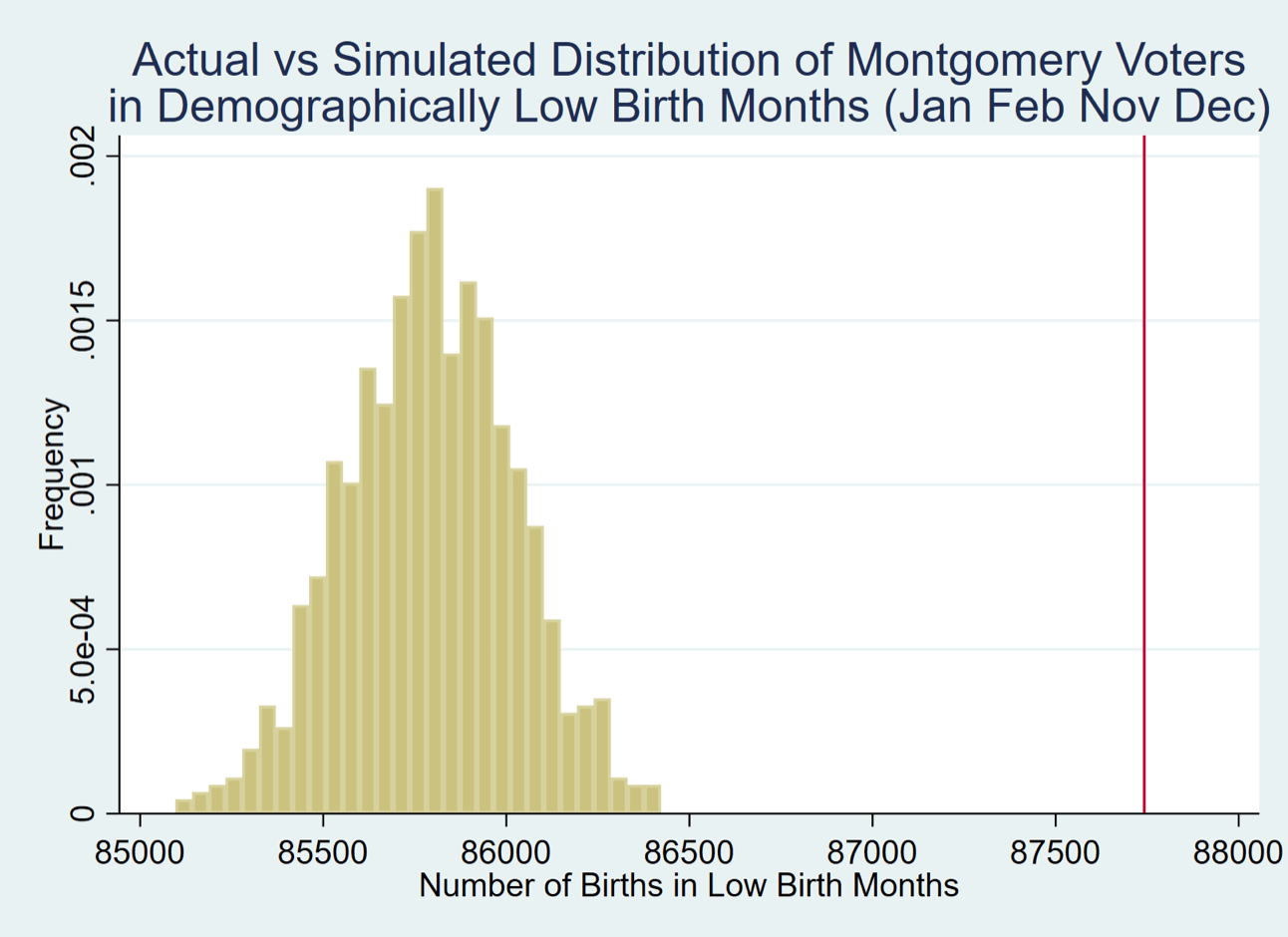
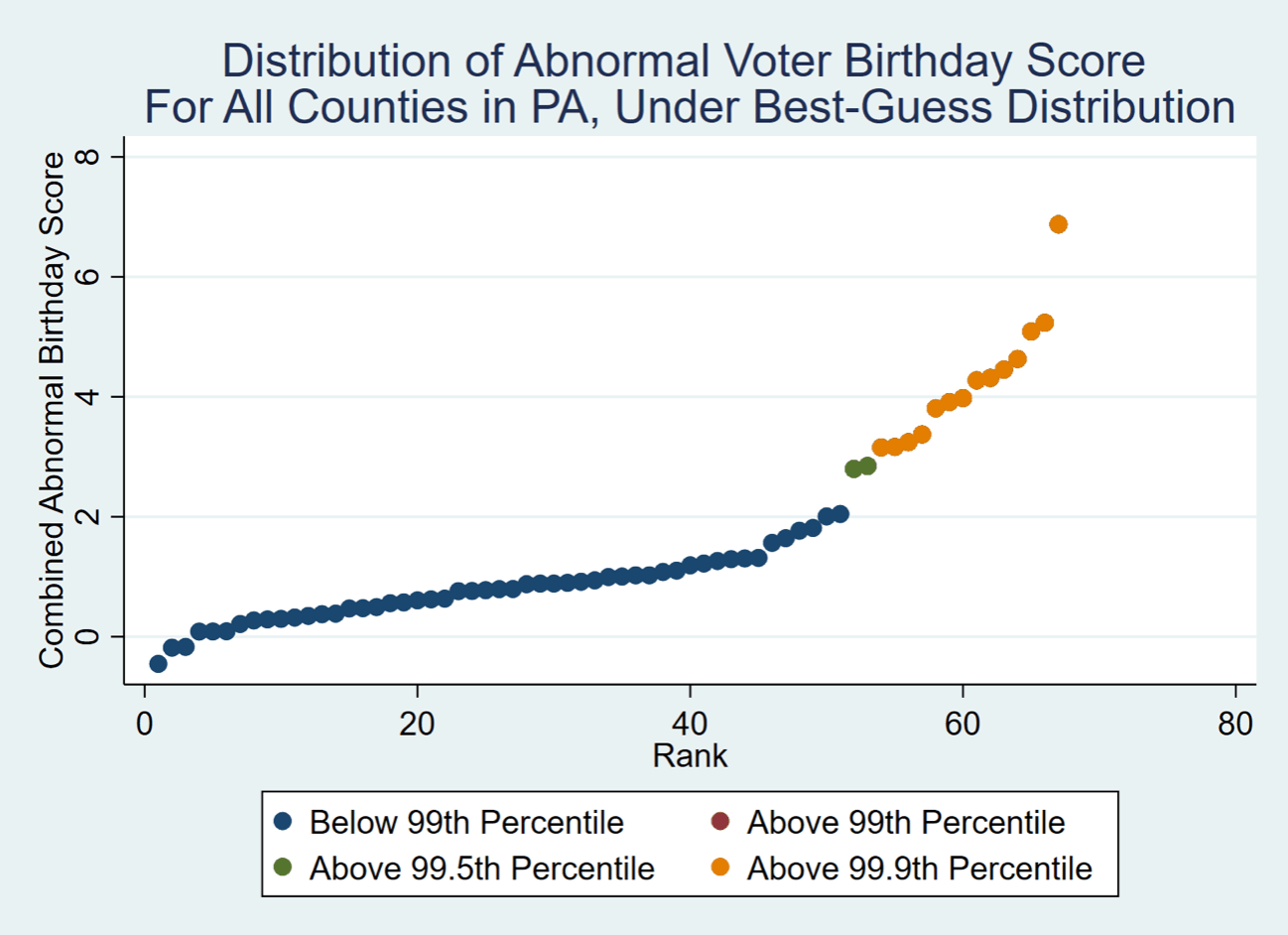
Under this metric, there are now 14 counties that are above the 99.9th percentile of the simulated distribution.
Relation between Abnormal Birthdays and Biden Vote Share
SNext, we consider how these measures of abnormal birthdays may have impacted the election. We first examine the relationship between abnormal birthdays and the two-party vote share for Biden.
The easiest way to visualize this is just with a scatter plot of the Biden two-party vote share and the percentile of abnormal birthdays.
To formally test the relationship, we regress the Biden two-party vote share on either the abnormal birthday z-score or percentile. Even with only 67 data points, the relationship is strongly statistically significant, with regression p-values for the main explanatory variable of abnormal birthdays being 0.000008 and 0.0002 respectively.
The size of the effect is also economically large – a one standard deviation increase in abnormal birthday z-scores under the conservative benchmark (1.068) is associated with a higher Biden two-party vote share by 6.8 percentage points.
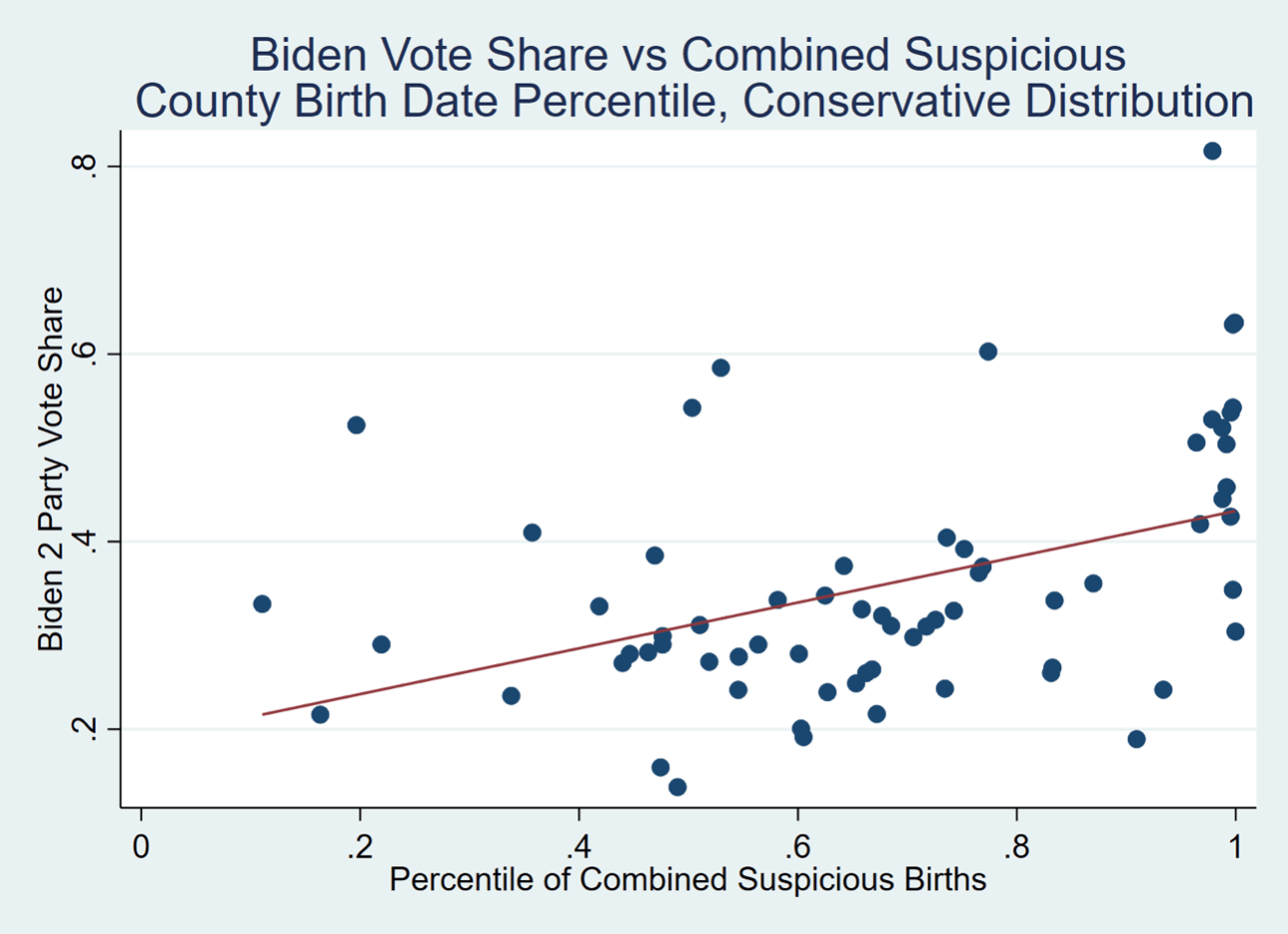
If the same graph is plotted for z-scores, the relationship is also evident.
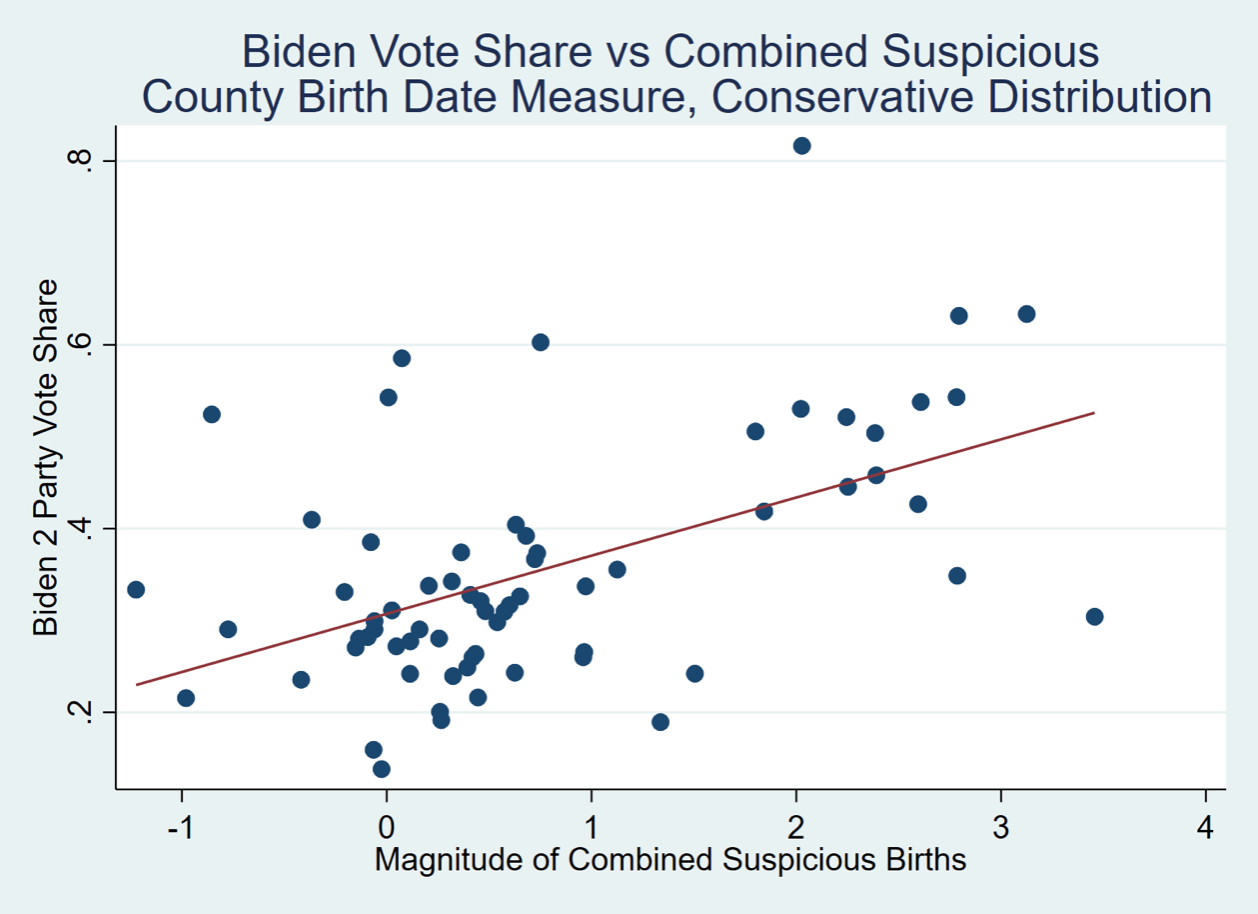
Relationship with Historical Democrat Vote Share
In addition, we would like to test how suspicious birthday distributions relate to a stronger measure – the difference between Biden’s two-party vote share and the historical performance of Democratic presidential candidates in the same county. Using the MIT Election lab data, we consider all presidential elections since 2000, and take the maximum Democratic vote share in that county across all five previous elections. This is now ensuring that the high Biden vote share associated with abnormal birthdays is not just measuring counties that always vote for Democrats.
We run the same regressions as last time, but instead take as the dependent variable the difference between Biden’s two-party vote share, and the maximum Democrat two party vote share over the previous five presidential elections. Most of these observations are now negative, reflecting the fact that in any given observation, one is normally below the historical maximum.
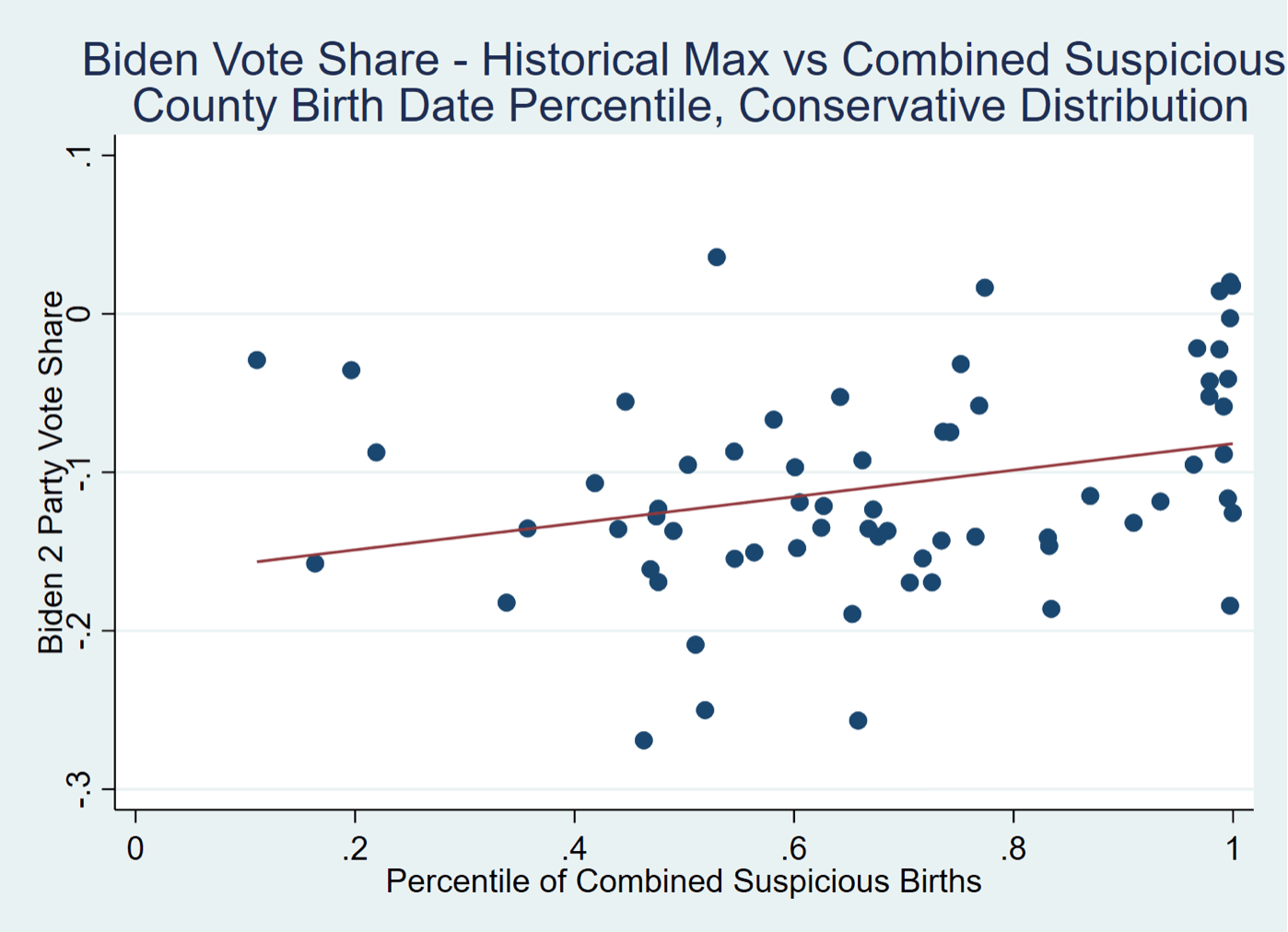
The relation is similar to before. Greater suspicious birthdays are also associated with higher performance for Biden relative to all elections since 2000. Once again, the relation is positive and highly statistically significant, with p-values of 0.0027 and 0.016 for regressions using the z-score and percentile respectively. In terms of magnitudes, a one standard deviation in the z-score is associated with a higher Biden vote share relative to historical elections by 2.4 percentage points.
Another way to show this result is the following. There are 5 counties out of 67 where Biden’s two-party vote share exceeded the performance of the Democratic candidate in all presidential elections since 2000 – Montgomery, Delaware, Cumberland, Allegheny, and Chester. Of these, three of them score above the 98th percentile of suspicious birthdays, and two score above the 99.7th percentile.
SEffect on Jorgensen/Trump Two Party Vote Share
Another secondary indication of fraud is that not only is Biden likely to gain relative to Trump, but in addition Jorgensen is likely to gain relative to Trump. The reason is that any fraud perpetrator targeting the statewide race wants to simultaneously add as many votes as possible to Biden, and add as few as possible to Trump (since each Trump vote erodes the margin they’re trying to add to). However, reporting an enormous overall vote for Biden is likely to look suspicious. As a consequence, another way to transfer net two-party margin to Biden is to also increase the votes for the Libertarian candidate.
We test this using the same methods as before, but instead examining the Jorgensen / Trump two party ratio. We find that greater levels of suspicious birthdays are also associated with a significantly higher vote share for Jorgensen relative to Trump. The p-value associated with abnormal birthdays in the regression is 0.00063 and 0.0021 for the versions using the z-score and the percentile measure, respectively.
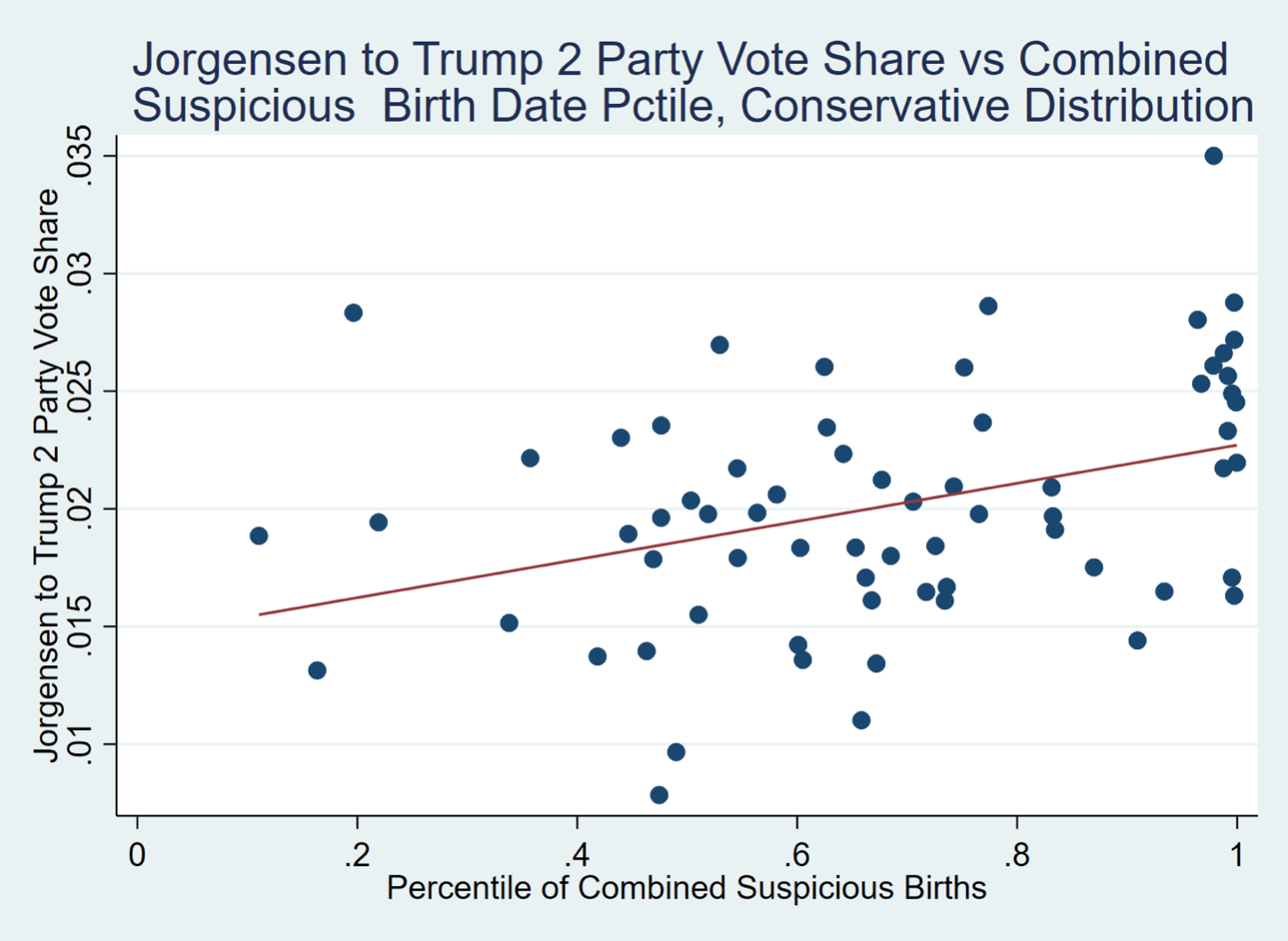
Overall Effect on Pennsylvania Election Results
Finally, we can use these results to estimate the likely effect of suspicious birthdays on the overall Pennsylvania election outcome. Because someone making up birthdays will not always select e.g. round days in January or December, the actual numbers of excess suspicious birthdays are likely to considerably understate the magnitude of possible fraud.
To estimate the magnitude of these effects, we use the relationship between excess birthdays and Biden vote share to estimate the effect of a change in the magnitude of suspicious births on county vote outcomes. In essence, we assume that the overall relationship between suspicious birthdays and Biden votes is due to voter fraud, and estimate how large a change in suspicious birthdays would be predicted to produce.
In particular, we consider what would happen if the eleven counties who scored above the 98th percentile of suspicious birthdays under the conservative distribution were instead to merely be exactly at the 98th percentile. This proceeds in three steps:
- Take the z-scores for each county, and work out how much lower they would be to get to the 98th percentile (a z-score of 2.095)
- Multiply this by the coefficient from the regression of Biden two-party vote share on the z-scores (0.0633). This then works out how much the two-party vote share would have changed
- Multiple this by the total number of two party votes in that county to get the overall change in Biden votes and Trump votes
We take the set of counties with z-scores above the 98th percentile (Northumberland (3.46), Delaware (3.13), Montgomery (2.79), Lawrence (2.79), Dauphin (2.78), LeHigh (2.61), Luzerne (2.60), Berks (2.39) Northampton (2.38), Cumberland (2.25) and Bucks (2.25)), and compute how many two-party votes would change sides if those counties were merely at the 98th percentile (2.095). This would change the total two party votes by 76,585. Since these would be votes being added to Trump and subtracted from Biden, this would be enough to overcome the 81,660 vote lead of Biden, and put Trump in the lead by 71,509 votes.
Conclusion
These patterns overall present strong evidence of two important facts about voter fraud in Pennsylvania. First, the distribution of birthdays in a number of counties significantly overweights unusual dates. Second, these counties look very suspicious along a large number of metrics and benchmarks, even when making deliberately conservative assumptions. Third, and independent of all the above, these patterns in birthdays are strongly associated with greater vote share for Biden, including at historically anomalous levels. Fourth, the magnitude of these deviations is large enough on its own to change the outcome of the Pennsylvania election, without reference to any of the other documented anomalies in Pennsylvania election data. Fifth, it is striking that Montgomery County, which was the subject of an entirely separate analysis of fraud, also looks strikingly suspicious along this alternative metric that has absolutely nothing to do with the variables previously studied. If one thinks that the previous analysis was just explained by data errors, one now has an entirely separate set of governmental data and separate tests that also confirm the same strong suspicions about fraud in the county. Nonetheless, this new metric allows the investigation to be considerably broadened, and finds evidence of fraud in other nearby large counties in Philadelphia. This raises serious questions about the integrity of the overall election result in Pennsylvania.
Carl Bell holds a Ph.D. in a quantitative discipline and works in a field relevant to statistical analysis.
Revolver News is dedicated to news aggregation and analysis. We are dedicated to providing Americans of all backgrounds and political persuasions with timely, common-sense, accurate and compelling information. Be sure to check out our news feed.
Please be aware that although we do not like to censor comments, we reserve the right to remove any that are uncivil, vulgar, or completely off-topic.
Join the Discussion